Editor’s Note: Anyone who reads blogs or newspapers is aware that big data is a hot topic for all industries. Unfortunately, the press (including well respected news organizations) seems to be confusing big data with analytics. As the editor of SourceCon I receive multiple articles each week that refer to big data. Like the press, these authors seem to all have a different idea of what big data is. In this post, Glen Cathey identifies the key distinctions between analytics and big data.
The interest in leveraging big data, analytics, and Moneyball in HR and recruiting is gaining significant steam.
Ever since my first article on the subject back in 2011, I’ve set up Google Alerts and Hootsuite streams set up to catch any mention of big data, analytics and/or Moneyball in conjunction with HR, sourcing or recruiting, and the volume of activity is bordering on surprisingly massive and overwhelming, and I’m not the only person to notice this.
Yes, it does seem like everyone is talking about big data in HR.
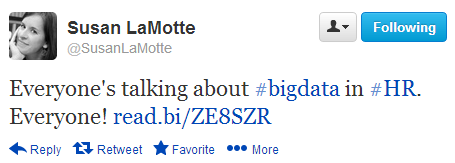
In 2012, “big data” was mentioned in 2.2M tweets by 980,000+ authors, at a peak rate of 3,070 times per hour!
However, as is often the case with relatively new and nebulous concepts, there is quite a bit of confusion surrounding big data and Moneyball and how they can be applied to HR and recruiting, as evidenced by the obviously incorrect usage of the terms in many cases. It’s also nearly impossible to stay on top of all of the content being generated on the subject (although I am trying my best!).
This is precisely why I’m going to take the opportunity to clear up any confusion by concisely explaining the concepts of big data, analytics, and Moneyball as it relates to HR and recruiting, as well as illustrate some obviously incorrect references to these concepts in recent articles, including those from the Wall Street Journal, Forbes, The Economist, The New York Times, and more.
I’ll tackle analytics first, big data second, and then Moneyball in HR/recruiting, leveraging Slideshare presentations and YouTube videos from experts for support.
Analytics ? Big Data!
Many articles use the term “big data” when they are really referring to analytics and data-based decision making.
For example, the recent New York Times article Big Data, Trying to Build Better Workers, The Wall Street Journal’s How Big Data Is Changing the Whole Equation for Business, and Forbes’ Big Data in Human Resources: Talent Analytics Comes of Age all use ”Big Data” in their titles, but the data they refer to do not meet the criteria for “big data” (definitively defined in a moment). The same can be said for the very popular Robot Recruiters article in The Economist.
Although inaccurate with regard to referencing “big data,” what each of those articles does do a good job of is provide excellent and interesting examples of leveraging analytics for HR/recruiting.
For example, Forbes’ piece features a company who ran a statistical analysis of sales productivity and turnover against a variety of demographic factors to develop a new sourcing and screening process to identify and hire people based on factors that data proved to be highly correlated with success, increasing sales performance by $4M in 6 months!
Analytics refers to the discovery and communication of meaningful patterns in data, which can be achieved with any data set, “big” or small.
Using analytics in human resources, such as developing correlations between employee performance, retention, demographic and assessment data to make data-based decisions is certainly a best practice, but making data-based decisions doesn’t have anything to do with “big data” unless the data being analyzed meets certain criteria.
Let’s explore the criteria required for data to be classified as “big data.”
So What is Big Data?
You may think you have a good grasp of the concept of big data, but if the misuse of the term by the writers for the New York Times, Forbes and many other respected publications is any indication, there is still quite a bit of confusion as to exactly what “big data” is and what it is not.
To clear up any confusion, I’ll guide you through the concept of big data, referencing industry pioneers and experts, as well as providing practical examples.
Wikipedia claims that “Big data is a term applied to data sets whose size is beyond the ability of commonly used software tools to capture, manage, and process the data within a tolerable elapsed time,” and that ”Big data sizes are a constantly moving target currently ranging from a few dozen terabytes to many petabytes of data in a single data set.” Other sources attempting to define big data include “the tools, processes and procedures allowing an organization to create, manipulate, and manage very large data sets…”
Regardless of how big data is defined or measured (terabytes, exabytes, etc.), the big data concept centers around relatively large amounts of data that are not only increasing in volume, but also in velocity and variety. Many big data pioneers and experts such as IBM agree that for something to be defined as “big data,” it should adhere to the “3 V’s:” Volume, Velocity, and Variety.
Here is IBM’s explanation of the 3 V’s along with some practical examples:
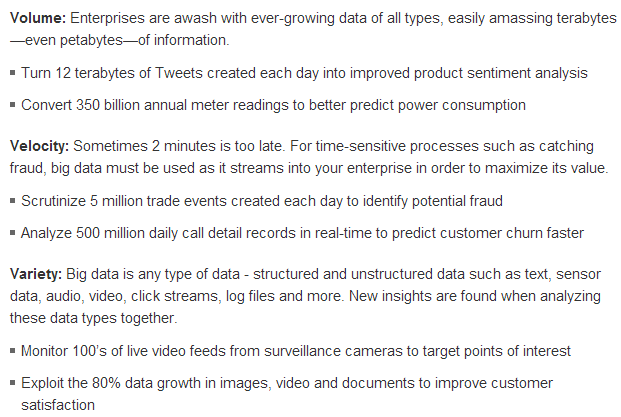
This excellent Slideshare containing a presentation on big data from Nick Weir, former VP of Data Strategy for Yahoo, clearly explains the “3 V’s” and is definitely worth a review.
Furthermore, Gartner’s definition of big data also includes the 3 V’s:
“Big data” is high-volume, -velocity and -variety information assets that demand cost-effective, innovative forms of information processing for enhanced insight and decision making.
When it comes to volume, the line seems to be drawn as low as the level of 100?s of gigabytes, but more often at the multi-terabyte+ level.
The variety aspect of big data refers to the mix of data types and sources (e.g., tracking sensors on employees) and varying degrees of structure, from structured to completely unstructured (free text in the form of social network updates, recommendations, awards, endorsements, blog posts, comments, press releases, announcements, etc.).
The “velocity” of data is the speed at which new data is generated. Social media provides and excellent example of high data velocity, with Twitter serving as the poster child, with over 400,000,000 tweets/day (that’s 2.8 billion updates every week!).
To get a better sense of how fast data (text, in this case) is being generated on Twitter, you can view real time Twitter activity via Tweetping (this is really cool!):
It really isn’t the volume of data that poses the processing challenge and requires the specific technologies that Gartner suggests – companies have been processing large volumes of data for over 10 years now, with massive data warehouses (50-100TB+) powering business intelligence, reporting, and analytics.
As you might imagine, it’s really the variety and velocity aspects of big data that necessitate the use of specialized information processing solutions. and more specifically unstructured data that poses the technology challenge.
So, to recap, no matter how large the data set being used to power analytics and to develop insights, if it doesn’t fit the 3 V criteria, it isn’t “big data” – the velocity and variety factors must also be present.
Now that we’ve established that understanding, get ready for this revelation: regardless of volume, velocity and variety, the value of big data isn’t the data!
Using technology to crunch big data is great, but the latent power of data lies in the ability to draw actionable insights (aka – analytics).
Check out this interesting presentation from Daniel Tunkelang, who currently serves as the Head of Query Understanding at LinkedIn (how’s that for a cool job?). He observes that data scientists worry about volume, variety and velocity, but the real bottleneck isn’t technology or computational – it’s cognitive!
Furthermore, powerful insight comes from a comment on the recent HBR article The Value of Big Data isn’t the Data, in which a data scientist with a Ph.D in machine learning recognizes the fact that we need business domain experts to ask the right questions:
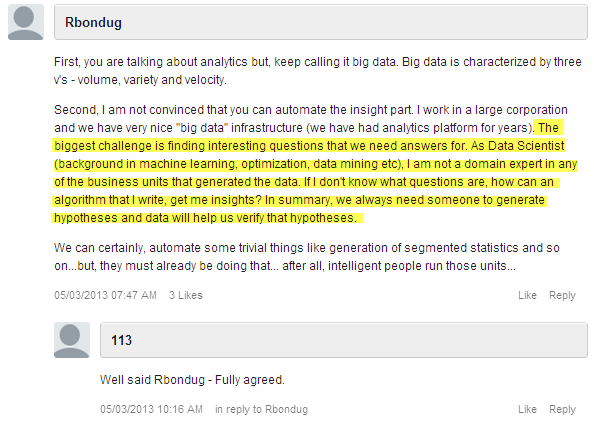
In other words, having a team of talented data scientists using all of the right technology isn’t enough to develop a competitive advantage.
Domain experts are necessary when building teams to develop big data insights and drive data-based decision making. When it comes to human resources and workforce science, the domain experts are HR professionals, sourcers, recruiters, and hiring managers – these are the people who should be able to ask the right questions that the data scientists can develop answers/solutions for.
Questions such as:
- Where do our best employees come from? (specific schools, companies, industries, etc.)
- What is the “DNA” of our best employees? (degrees, prior experience, backgrounds, demographics, personality traits, interests, etc.)
- How can we more effectively and consistently find and recruit our ideal employee profile?
- Who are our best managers?
- Do we really need to hire people with prior industry experience?
- Should we biased against “job hoppers?”
- How can we leverage assessments to increase our quality of hire?
- Does our interview process really “work?”
- Do reference checks actually have any value?
- Who should I be giving new challenges to/promoting?
- Who is likely to quit in the next 6 months?
- Where are our talent gaps today, and what will they be in near future?
- What are our most effective sources of talent, and why?
The Ideal Big Data /Analytics Team
I’ve marked up this excellent big data / big analytics graphic from Karmasphere to show examples of the types of relevant human capital data (structured and unstructured) that can be leveraged by various domain/functional experts – sourcers, recruiters, HRIS analysts, and hiring managers.
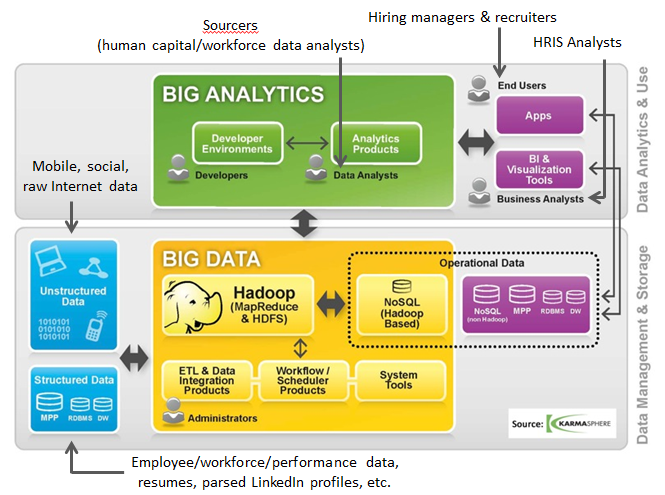
Additionally, this recent big data article in the Financial Times recognizes that the challenge we face when it comes to leveraging big data isn’t technology, it’s skills.
While there are many articles and experts that recognize the current and looming significant future talent shortage of analytics/data science professionals, I don’t agree with the idea that data scientists need or should be “all-in-one” technical, functional, and business experts – it is highly unlikely that all of these skills will be present in any one individual.
In fact, I think it’s ridiculous to expect data scientists to be able to ask all of the right questions, and the HBR article comment by the Fortune 500 data scientist with a Ph.D in machine learning confirms it.
Data scientists should be leveraged to crunch, analyze and present data to enable teams to derive sensible answers and possible solutions/courses of action based on questions developed by the functional/domain/business experts.
In software development, most mature software engineering departments don’t expect their software engineers to be “all-in-one” technical, functional, and business experts. Instead, software engineers rely on business analysts who serve as functional experts to solicit requirements from domain experts and users and translate them into technical specs and design documents that they pass on to software engineers to develop the solution.
Similarly, I believe the ideal talent analytics team is a mix of data scientists, human capital data analysts (sourcers), and domain experts (hiring managers, HR and recruiters). In fact, it could be argued that hiring data scientists with little-to-no specific business domain experience might actually be more effective – the story behind Moneyball actually supports that argument (Paul DePodesta graduated from Harvard with a degree in economics).
Speaking of Moneyball, let’s take a look at this other hot buzzword that is often used incorrectly when it comes to HR and recruiting.
Moneyball
To understand and correctly use a moneyball reference, you first have to learn its origin.
If you’re not already familiar with the origin of the team “moneyball,” it comes from Moneyball: The Art of Winning an Unfair Game, a book by Michael Lewis about the Oakland Athletics baseball team, its general manager Billy Beane and his assistant Paul DePodesta. I recommend reading the book if you haven’t already, watch the movie – even if you’re not a baseball fan (I’m not), or at least watch this 2.5 minute Moneyball trailer on YouTube.
The premise of the book is that the collected wisdom of baseball insiders (including players, managers, coaches, scouts, and the front office) over the past century with regard to player selection is subjective and often flawed. The Oakland A’s didn’t have the money to buy top players, so they had to find another way to be competitive. Billy and Paul took an analytical, statistical and sabermetric approach to assembling their team, picking players based on qualities that defied conventional wisdom and the beliefs of many baseball scouts and executives.
For example, Billy Beane and Paul DePodesta found that on-base percentage and slugging percentage are better indicators of offensive success than batting averages, and the A’s became convinced that these qualities were cheaper to obtain on the open market than more historically valued qualities such as speed and contact. Billy and Paul also often picked players that other scouts and teams would overlook because the players didn’t have the right body type or they had a funny swing.e book is that the collected wisdom of baseball insiders (including players, managers, coaches, scouts, and the front office) over the past century with regard to player selection is subjective and often flawed.
The Oakland A’s didn’t have the money to buy top players, so they had to find another way to be competitive. Billy and Paul took an analytical, statistical and sabermetric approach to assembling their team, picking players based on qualities that defied conventional wisdom and the beliefs of many baseball scouts and executives.
Seriously!
Check out this YouTube video in which Paul DePodesta is talking about recruiting by body type and this one in which a team loses out on a future hall of fame player because someone thought he had “the weakest freaking hack” they had ever seen.
In 2002, with approximately $41 million in salary, the Oakland A’s were competitive with larger market teams such as the New York Yankees, who spent over $125 million in payroll that same season. The A’s finished 1st in the American League West and set an AL record of 20 consecutive wins. Today, many professional baseball teams employ sabermetricians, and in fact, the NY Yankes now employee a whole team of sabermetric analysts.
Ultimately, the essence of “moneyball” lies in using data and statistics to “arbitrage miscalculated pay rates” (those are Billy Beane’s exact words from a keynote I recently attended), to avoid overvalued skills/experience, and to identify undervalued skills when building teams to develop a competitive advantage without having to “buy” expensive talent. If you are leveraging data to identify and acquire people who can perform the same or better than people who are more expensive to acquire, then the moneyball concept applies (hence the “money” in moneyball).
For example, if you can find, hire and train a certain type of person with no prior specific industry or role experience, who, once trained, can outperform people in the same role who have 5-10 years of specific role/industry experience, then you are effectively “playing” moneyball. I’ve been doing this for years with sourcers, recruiters and sales people.
Why pay to hire someone with 10 years of experience when the right person with less or no experience can outperform them at a fraction of the cost?
Moneyball in HR/Recruiting
Now that you have a clear understanding of the moneyball concept, you can appreciate that articles such as Moneyball in the Workplace, Forbes’ What Moneyball Can Teach You About Hiring the Right People, and Business Insider’s MONEYBALL AT WORK: They’ve Discovered What Really Makes A Great Employee aren’t actually about moneyball at all.
While one of the lessons in Moneyball is to challenge conventional wisdom, there has to be some aspect of labor arbitrage at work or else there is no money in the moneyball approach.
Business Insider’s “Moneyball” article talks quite a bit about what really makes a great employee, and many of the findings illustrated do fly in the face of conventional hiring wisdom, there isn’t any aspect of identifying and acquiring the same level of talent at a lower cost, or better talent at the same cost. The Business Insider article also incorrectly references big data, which as I’ve noted above is quite common.
What the MONEYBALL AT WORK: They’ve Discovered What Really Makes A Great Employee article does do a good job of, however, is illustrate excellent, practical and real-world examples of leveraging data to develop non-intuitive and actionable insights into what makes a great employee – aka talent analytics or workforce science. Many “moneyball” articles reference Evolv On Demand’s work, including findings such as:
- People who fill out online job applications using browsers that did not come with the computer (such as Microsoft’s Internet Explorer on a Windows PC) but had to be deliberately installed (like Firefox or Google’s Chrome) perform better and change jobs less often
- For customer-support call center jobs, people with a criminal background actually perform a bit better than people who don’t have a criminal background
- Call center workers who had “job-hopped” in the past were no more likely to quit quickly than those who had not frequently changed jobs
These are all excellent examples of analytics / workforce science, but not moneyball, unless the insights are leveraged for a combined cost/talent advantage. In that vein, here is a solid moneyball example from Evolv, demonstrating their finding that, for hourly workers, it is not necessary to pay top dollar for experienced labor because people with no previous experience in a similar job had the same “probability of survival” over 180 days as experienced workers.
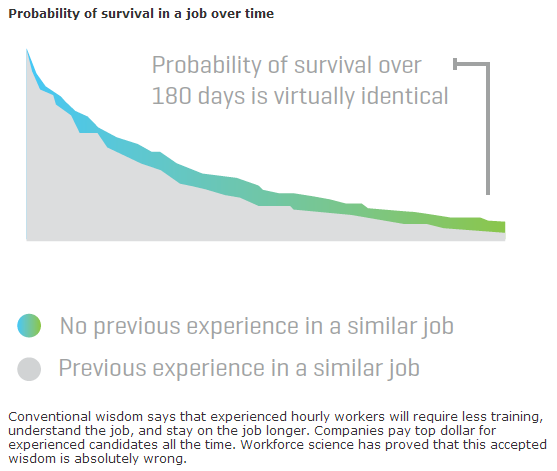
The reason why this can be used as an accurate reference to Moneyball is because the findings can allow companies to hire from a lower cost labor pool and achieve the same business results.
Evolv has been performing some very interesting work – if you haven’t already, I recommend you download their Q2 2013 Workforce Performance Report.
Although it incorrectly makes reference to big data, if we revisit the Forbes article Big Data in Human Resources: Talent Analytics Comes of Age, one of the examples demonstrated within could be a good example of moneyball. One of Bersin by Deloitte’s clients, a large financial services company, believed that their best sales people came from prestigious universities and achieved high G.P.A.’s. One of their analysts “performed a statistical analysis of sales productivity and turnover. They looked at sales performance over the first two years of a new employee and correlated total performance and retention rates against a variety of demographic factors.”
Here is what they found:
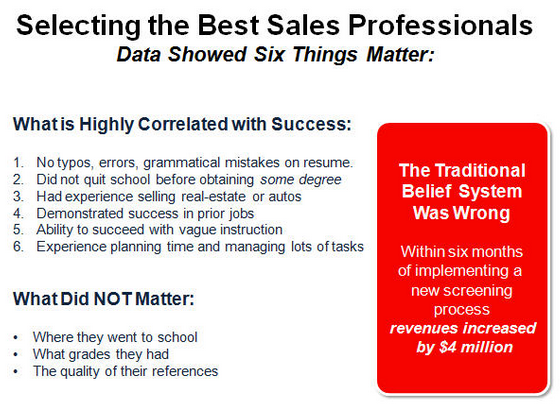
While this is not an example of “big data,” it is a powerful example of analytics and moneyball – they were able to use data to challenge their own conventional wisdom when it came to hiring sales professionals, and to change their sourcing and screening process to identify and hire people based on factors that data proved to be highly correlated with success, and increase their performance ($4M in 6 months!) as a result.
While not explicitly stated in the article, one could presume that they could (and probably did) lower their cost per hire by not targeting people coming from prestigious universities who might command higher compensation.
Analytics, Big Data and Moneyball for HR/Recruiting – Take Aways
I’ve covered a lot of ground in this article, but I can summarize the main points quite concisely:
- Analytics is the discovery and communication of meaningful patterns in data, which can be achieved with any data set – big or small. You don’t need big data to leverage analytics and drive data-based decision making
- No matter how “big” the data set, if it doesn’t meet the 3V criteria – volume, velocity, variety – it isn’t “big data”
- The foundation of the Moneyball concept is based on using data, statistics and analytics to achieve a form of labor arbitrage – to identify undervalued skills when building teams to develop a competitive advantage without having to “buy” expensive talent. If you’re only using data and analytics to challenge conventional wisdom, it doesn’t qualify as “moneyball” – there must be some aspect of cost savings and/or increased performance/results at the same cost
- Don’t expect data scientists to solve all of your talent challenges and develop data-based insights by themselves. The ideal analytics team is diverse and should involve domain experts to ask the right questions. When it comes to hiring, the domain experts are hiring managers, HR professionals and recruiters.
This post previously appeared on Glen’s blog, booleanblackbelt.com